Artificial intelligence continues to evolve rapidly, giving rise to AI-driven βagentβ frameworks that can autonomously handle tasks and communicate with APIs in a human-like manner. Unlike typical automation scripts, AI agents leverage large language models (LLMs) to interpret, learn, and make context-based decisions. They take on repetitive choresβlike analyzing new pull requests or generating release notesβso developers can focus on what truly matters.
Why AI Agents Over Traditional Automation?
Traditional automation scripts are often static, following predefined if-else rules or workflows. By contrast, AI agents adapt and refine their own instructions, making them more flexible. For example, an agent configured to detect potential security risks in PRs can learn from new vulnerabilities or updated code patterns, rather than being limited to a rigid set of checks.
Common Developer Tasks to Automate
- Pull request reviews: Summarize diffs, catch style issues, and highlight security risks.
- Release notes: Parse commit messages for automatically generated release content.
- Code reviews and triaging: Suggest improvements, identify best practice violations, and group user-reported issues by root cause.
- Debug log analysis: Surface patterns in log data and proactively recommend pipeline optimizations or environment fixes.
Project Goals and Expected Outcomes
Set up an AI agent that responds to triggers like new commits and integrates with Semaphore’s CI/CD pipeline to automatically summarize and review the changes.
Setting Up the Project and Choosing Your AI Agent Tools
Key Tools and Frameworks
You have several options for building an AI agent:
- AgentGPT & AutoGPT: Out-of-the-box βautonomousβ GPT-driven frameworks.
- LangChain: Comprehensive toolkit for chaining LLM prompts and external APIs.
- LlamaIndex: Data-centric approach to building context/query structures on top of LLMs.
- Vercel AI SDK: Streamlined developer experience with built-in tooling for prompt orchestration, plus integration with providers like OpenAI.
Each solution has strengths: AgentGPT/AutoGPT for faster prototypes, or lower-level libraries like LangChain and the Vercel AI SDK for robust customization.
Version Control, Plugins, and Security Measures
Keep your AI agent code in a dedicated repository or a separate folder in your main codebase. Adhere to best practices such as branching for feature work, small commits, and meaningful commit messages. This ensures that your automated tasks remain transparent and easy to maintain.
Whether youβre linking to GitHub, Slack, Jira, or other services, store credentials securely (e.g., in Semaphore secrets). Give your AI agent the minimal required permissionsβlike read-only on certain repos or specific Slack channelsβso you donβt compromise security.
Use caution when your AI agent interacts with external services:
- Store tokens securely (e.g., environment variables in secrets).
- Review data exposuresβespecially in logs.
- Rotate tokens and credentials regularly.
Defining and Automating Daily Developer Tasks
Identifying Tasks and Designing Task Workflows
List out routine tasks that could benefit from LLM-powered automation:
- Code scanning (security, style checks)
- Creating draft release notes
- Debugging suggestions from logs
- Summarizing PRs, especially large ones
- Triage and labeling of new GitHub issues
Define the input and output requirements:
- Clear triggers like pushing a commit or a tag
- Well-defined outputs like AI-generated summaries, posted comments, or updated Slack messages
Define consistent error handling:
- Retry with exponential back-off
- Notify a Slack channel or email list for manual intervention
Implementation Details
Code Structure:
- Use a logical folder structure.
- This makes it easier to add or refine tasks without confusion.
Logging and Data Storage:
- Agents often produce valuable metadata such as logs of LLM calls, error messages, or final decisions.
- Decide on a storage approach (like local JSON logs or a time-series database) that your team can later review or analyze for improvements.
Code Reviews and Commit Summaries
In this step, we’ll explore a small Node.js agent powered by the Vercel AI SDK that automatically reviews commits, summarizes code changes, and flags security or style issues. The goal is to reduce manual overhead and let developers focus on high-impact work rather than repetitive, preliminary checks.
Benefits and Key Objectives for Commit Agent
- Automated Commit Triggers
- Integrate your AI agent with GitHub’s webhooks or rely on Semaphore’s pipeline triggers whenever a new commit is pushed.
- This ensures immediate detection of code changes and helps your team fix problems at the earliest possible time.
- Intelligent Summaries & Security Checks
- By leveraging GPT-4 or other LLMs, your AI agent can parse diffs and highlight potential vulnerabilities, style problems, or major architectural changes.
- Reviewing a large commit becomes easier when a summarized outline is readily available.
- Actionable Feedback & Suggestions
- Developers can receive direct improvement tipsβfrom refactoring opportunities to adopting best practices for dependency management.
- These suggestions reduce the mental overhead required during code review.
- Seamless CI/CD Integration
- With the help of Semaphore blocks and jobs, you can automatically run the code review agent removing the need for manual triggers.
- Comments or logs can be posted to PR threads enabling quick, iterative feedback loops.
Getting the Project
To access the code review agent, clone the repository:
git clone git@github.com:ajcwebdev/semaphore-ai-agents.git
cd semaphore-ai-agents
Project Dependencies
The project uses the following key dependencies:
- aiΒ andΒ @ai-sdk/openaiΒ – The core Vercel AI SDK and OpenAI provider packages
- zodΒ – Helps define schemas for validating or structuring data
Environment Setup
The project requires a .env
file with the following variables:
OPENAI_API_KEY=YOUR_OPENAI_KEY
GITHUB_TOKEN=YOUR_GITHUB_TOKEN
GITHUB_OWNER=YOUR_GITHUB_USERNAME
GITHUB_REPO=YOUR_GITHUB_REPO
For example, if I want my AI Assistant to review this codebase ajcwebdev/semaphore-ai-agents, I’d set GITHUB_OWNER=ajcwebdev
& GITHUB_REPO=semaphore-ai-agents
. If you’ve forked the repo, you will have the same GITHUB_REPO
name but your own GITHUB_OWNER
account name.
You’ll need to create a fine-grained personal access token on GitHub at github.com/settings/personal-access-tokens. The only permission required is read-only access to the public GitHub repository that is connecting to Semaphore.
Understanding the Code Structure
The overall project structure looks like this:
semaphore-ai-agents/
βββ .env
βββ .gitignore
βββ package.json
βββ src/
β βββ config.ts
β βββ index.ts
β βββ ai/
β β βββ review.ts
β βββ commits/
β β βββ parser.ts
β βββ github/
β βββ tools.ts
βββ .semaphore/
βββ semaphore.yml
Let’s examine the key components of the commit analysis agent:
1. Configuration Module (src/config.ts)
This module manages environment variables and validation. The code loads environment variables and provides a validation function to ensure all required configuration is present before proceeding.
2. GitHub API Tools (src/github/tools.ts)
This module handles GitHub API interactions.
This module provides two key functions:
fetchCommitDiff
Β – An AI SDK tool that retrieves the diff for a specific commit SHAfetchCompareCommits
Β – A function that fetches all commits between two SHAs using GitHub’s compare API
3. Commit Parser (src/commits/parser.ts)
This module handles parsing commit ranges. The code intelligently resolves the commit ranges provided by Semaphore CI environment variables. It handles both:
- Single commits (when onlyΒ
SEMAPHORE_GIT_SHA
Β is available) - Commit ranges using GitHub’s compare API (whenΒ
SEMAPHORE_GIT_COMMIT_RANGE
Β is available)
It also converts two-dot notation (..
) to three-dot notation (...
) for compatibility with GitHub’s API.
4. AI Review Generator (src/ai/review.ts)
This module generates the AI-powered code reviews. The module uses the Vercel AI SDK to generate intelligent code reviews. It:
- Uses GPT-4o to analyze commit diffs
- Provides specific instructions to summarize changes, flag issues, and suggest improvements
- Uses theΒ
fetchCommitDiff
Β tool to retrieve the code changes for analysis - Limits execution to 2 steps to optimize performance
5. Main Entry Point (src/index.ts)
This is the main orchestration file that brings everything together. The main file coordinates the entire review process:
- Validates the environment configuration
- Resolves the commits to review
- Generates AI reviews for each commit
- Outputs the reviews to the console
- Handles errors properly to ensure CI failures are reported
Semaphore CI/CD Integration
Open the Settings page on your Semaphore account and select the “Secrets” tab to create pr-review-secrets
. Include the same environment variables inside your project’s .env
file.
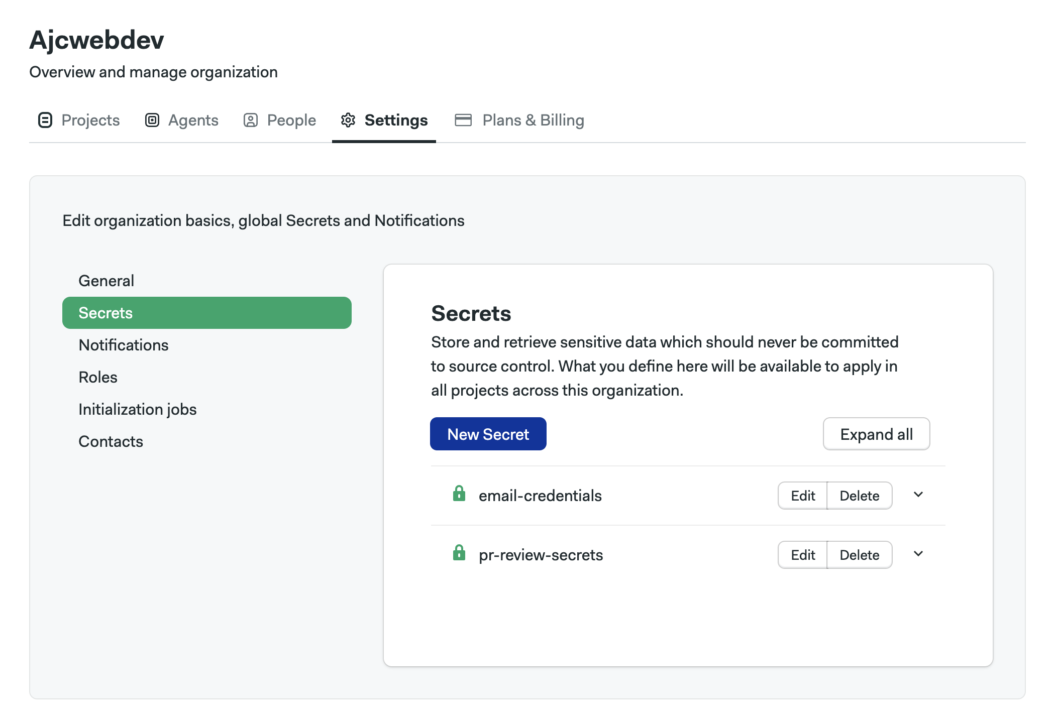
Semaphore Configuration File
The project includes a Semaphore configuration file to trigger the agent on new commits. The AI output is captured in a file called ai-review.log
and stored as an artifact for easy access in the Semaphore UI.
# .semaphore/semaphore.yml
version: v1.0
name: Code Review Pipeline
agent:
machine:
type: e1-standard-2
os_image: ubuntu2004
global_job_config:
prologue:
commands:
- checkout --depth=full
- npm install
blocks:
- name: Commit Review
task:
secrets:
# Create pr-review-secrets in the Semaphore UI or with the Semaphore CLI
- name: pr-review-secrets
jobs:
- name: Review Commits
commands:
# Run AI review script, capturing the output in a log file
- npx tsx src/index.ts | tee ai-review.log
# Push AI review log as a job artifact, view from Semaphore UI Artifacts tab.
- artifact push job ai-review.log
dependencies: []
This configuration:
- Sets up a build pipeline that runs on Ubuntu 20.04
- Checks out the full commit history to ensure proper diff generation
- Installs dependencies and runs the review script
- Saves the AI review output toΒ
ai-review.log
, then pushes it as aΒ job artifact - Uses a Semaphore secret namedΒ
pr-review-secrets
Β to securely provide the required environment variables
When Semaphore triggers a build on new commits, it will set SEMAPHORE_GIT_COMMIT_RANGE
(or SEMAPHORE_GIT_SHA
if only one commit is pushed). The script retrieves each commit’s diff and generates an AI review.
The review is stored both in the Semaphore logs (via tee
) and as an artifact (ai-review.log
). You can open the job log to read the review directly:
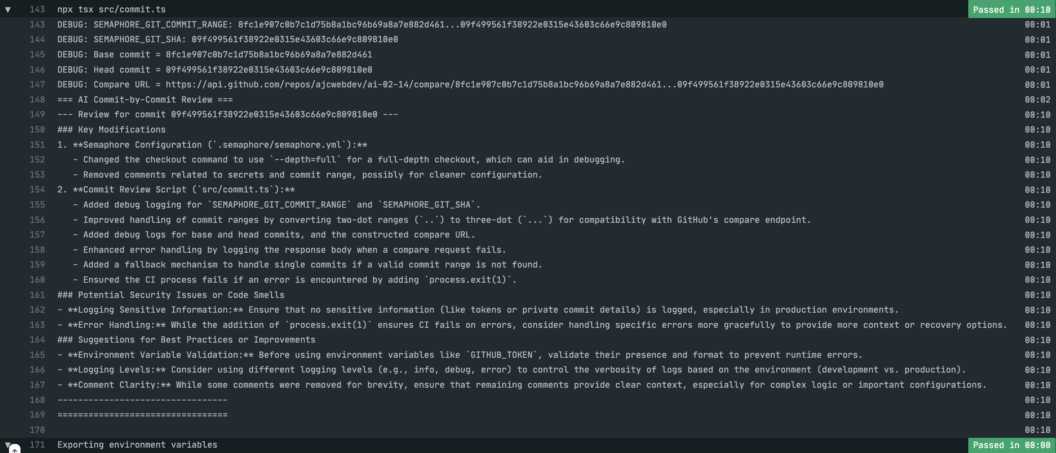
Or go to theΒ ArtifactsΒ tab in your Semaphore job page to download the file:
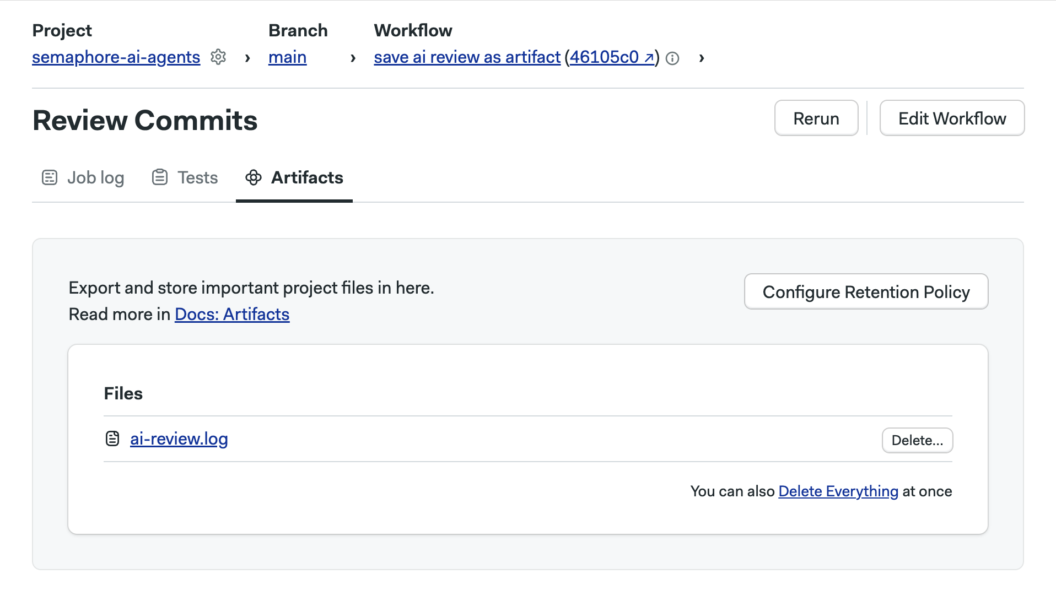
Benefits of This Modular Approach
The repository’s modular structure offers several advantages:
- Separation of concerns: Each file handles a specific aspect of the application
- Testability: Components can be tested in isolation
- Maintainability: Smaller focused files are easier to understand and modify
- Reusability: The modules can be reused across different parts of the application
By leveraging this AI-powered commit review agent, your team can gain immediate insights about each commit in a push or pull request, helping to identify potential issues before they reach human reviewers. Storing the artifact with the AIβs output makes it easy for the whole team to access and reference code changes.
Conclusion
Recap
We started by discussing the motivations behind AI agents and why they can surpass traditional, rule-based automation. We walked through the basics of setting up your project, selecting the right frameworks, and defining your automated workflows. Then we dove into how to build an AI agent for commit analysis that provides summaries, security checks, and style feedback.
Best Practices
- Prompt Engineering: Craft system messages and prompts that guide the LLM to produce actionable feedback.
- Secure Credential Management: Always store tokens and secrets in a protected environment, such as Semaphore’s secrets.
- Monitoring & Iteration: AI agents improve with continuous prompts, data logs, and examples. Regularly review their suggestions for accuracy.
- Limiting Permissions: Provide the minimal necessary accessβreduce the risk of unintentional modifications.
Next Steps & Future Improvements
- Advanced Analytics: Integrate more robust usage metrics and dashboards to refine the agent’s suggestions over time.
- Complex Integrations: Explore adding JIRA or Asana tasks automatically based on your agent’s analysis or code updates.
- Refined Prompting Strategies: Use conversation memory or chain-of-thought techniques for even more accurate agent responses.
- Support for Additional Developer Tools: Incorporate tools like ESLint, SonarQube, or custom security scanners for deeper code insights.
Call to Action
Clone or fork the example repository, add your own environment variables, and integrate the AI agent with your existing CI/CD pipeline on Semaphore. You’ll quickly see how these agents can reduce your overhead while improving code quality and release workflows.
Nice overview, thanks for sharing. Just wondering, between AgentGPT and LangChain, which one is more beginner-friendly for someone without a lot of AI background? This stuff looks impressive but a little intimidating at first.